MetricNet: A Loop Closure Detection Method for Appearance Variation using Adaptive Weighted Similarity Matrix
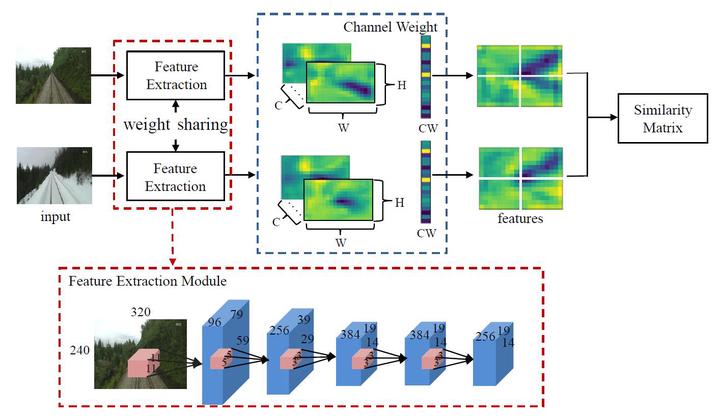
Background
Loop Closure Detection (LCD), also known as visual place recognition, provides extra information that robot has visited this area before. This constrain assists the positioning system to reduce the pose estimation error by following optimization algorithm.
Contribution
The proposed MetricNet contains two blocks: 1) feature extraction leveraging deep learning; 2) similarity measurement with adaptive weight. As an end-to-end loop closure detector, MetricNet is trained by minimizing the binary cross-entropy (BCE) consisting of label and calculated similarity score. The contributions can be summarized into:
- Effective feature selection and adaptive similarity matrix: The learning-based feature extraction exploits spatial information. Inspired by inverse documentary frequency (IDF), a channel weight mechanism is devised to distil features that are effective for image matching. In terms of similarity calculation, it considers the link between diagonal and off-diagonal elements of similarity matrix.
- Accurate and robust detection performance: Extensive experiments reveal that MetricNet outperforms both learning-based and conventional approaches, especially under the condition with distinct apperrance changes.